
The Rise of Neuro-Symbolic AI: A Paradigm Shift in Artificial Intelligence
Sep 19
4 min read
1
38
0
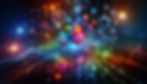
Neuro-Symbolic AI (NSAI) Arrives
In the rapidly evolving landscape of artificial intelligence, a new paradigm is emerging that promises to bridge the gap between traditional AI approaches and human-like reasoning. Neuro-Symbolic AI (NSAI) stands out as a revolutionary architecture that combines the strengths of neural networks with symbolic reasoning, offering a more robust and interpretable approach to AI. This discussion explores how NSAI differentiates itself from "Regular AI" architectures and its potential to reshape the future of artificial intelligence.
"Regular AI" Architectures Defined
Traditional or "Regular AI" architectures typically fall into two main categories: rule-based systems and machine learning models. Rule-based systems rely on predefined sets of rules and logic to make decisions, while machine learning models, particularly deep learning neural networks, learn patterns from vast amounts of data to make predictions or classifications.
These conventional approaches have achieved remarkable success in various domains, from image recognition to natural language processing. However, they often struggle with tasks requiring complex reasoning, adaptability to new situations, or transparent decision-making processes.
Neuro-Symbolic AI Defined
Neuro-Symbolic AI represents a hybrid approach that integrates neural networks' pattern recognition capabilities with symbolic AI's logical reasoning and knowledge representation. This fusion aims to create AI systems that can learn from data, reason about abstract concepts, and explain their decision-making processes in human-understandable terms.
At its core, NSAI combines the bottom-up learning of neural networks with the top-down reasoning of symbolic systems. This integration allows for more flexible and powerful AI models that can handle both structured and unstructured data while maintaining the ability to perform logical inference and reasoning.
How Does Neuro-Symbolic AI Stand Out from "Regular AI"?
Neuro-Symbolic AI distinguishes itself from traditional AI architectures in several key ways:
Interpretability and Explainability: Unlike black-box neural networks, NSAI models can provide clear explanations for their decisions by leveraging symbolic reasoning. This transparency is crucial for building trust in AI systems, especially in sensitive domains like healthcare and finance.
Read more about the "black box" problem: AI Transparency & the "Black Box" Problem: Neuromorphic vs. Neuro-Symbolic AI
Efficient Learning: NSAI can learn from smaller datasets by incorporating prior knowledge and logical rules. This efficiency contrasts with deep learning models that often require massive amounts of data to achieve good performance.
Reasoning Capabilities: By integrating symbolic logic, NSAI can perform complex reasoning tasks that are challenging for pure neural network approaches. This includes causal reasoning, analogical thinking, and abstract concept manipulation.
Flexibility and Adaptability: NSAI architectures can more easily adapt to new situations by combining learned patterns with logical rules, making them more versatile in real-world applications where conditions may change rapidly.
Knowledge Integration: NSAI allows for the seamless integration of domain knowledge and expert insights into the AI system, enhancing its performance and reliability in specialized fields.
Real-World Applications
The potential applications of Neuro-Symbolic AI are vast and diverse:
Healthcare: NSAI can assist in medical diagnosis by combining pattern recognition from medical images with logical reasoning based on patient history and medical knowledge bases. This approach can lead to more accurate and explainable diagnoses.
Financial Services: In risk assessment and fraud detection, NSAI can analyze transaction patterns while applying complex regulatory rules, providing both high accuracy and clear justification for decisions.
Autonomous Vehicles: NSAI can enhance decision-making in self-driving cars by combining learned driving behaviors with explicit traffic rules and ethical considerations.
Natural Language Understanding: By integrating semantic knowledge with neural language models, NSAI can achieve deeper comprehension of text, improving tasks like question answering and content generation.
Robotics: NSAI can enable robots to learn from experience while adhering to safety protocols and logical constraints, making them more adaptable and reliable in diverse environments.
Future Development & Challenges
While Neuro-Symbolic AI shows great promise, several challenges need to be addressed for its widespread adoption:
Integration Complexity: Seamlessly combining neural and symbolic components remains a significant technical challenge, requiring innovative architectural designs and learning algorithms.
Scalability: As NSAI systems grow in complexity, ensuring their scalability to handle large-scale real-world problems becomes crucial.
Knowledge Representation: Developing efficient and flexible methods for representing symbolic knowledge that can interact effectively with neural components is an ongoing area of research.
Balancing Learning and Reasoning: Finding the right balance between data-driven learning and rule-based reasoning to optimize performance across various tasks is a key challenge.
Ethical Considerations: As NSAI systems become more capable of complex reasoning, ensuring they align with human values and ethical principles becomes increasingly important.
Despite these challenges, the future of Neuro-Symbolic AI looks promising. Ongoing research is focusing on developing more sophisticated integration techniques, improving the scalability of NSAI systems, and exploring novel applications across various industries.
Conclusion
Neuro-Symbolic AI represents a significant leap forward in the field of artificial intelligence, offering a bridge between the pattern recognition capabilities of neural networks and the logical reasoning of symbolic systems. By addressing the limitations of traditional AI architectures, NSAI opens up new possibilities for creating more intelligent, interpretable, and versatile AI systems.
As research in this field progresses, we can expect to see NSAI playing an increasingly important role in solving complex real-world problems that require both learning from data and reasoning about abstract concepts. The potential impact of Neuro-Symbolic AI extends across numerous sectors, from healthcare and finance to robotics and beyond, promising to bring us closer to AI systems that can truly emulate human-like intelligence.
The journey towards fully realized Neuro-Symbolic AI is still in its early stages, but the potential benefits are immense. As we continue to refine and develop this hybrid approach, we move closer to creating AI systems that not only match but potentially surpass human capabilities in complex reasoning tasks while maintaining transparency and interpretability. The future of AI is not just about smarter machines, but about machines that can think, reason, and explain their decisions in ways that align with human understanding and values.
Citations:
https://dig8italx.com/what-is-ai-architecture-important-things-you-need-to-know/
https://www.sdxcentral.com/artificial-intelligence/definitions/what-is-artificial-intelligence/
https://www.deltek.com/en/innovation/ai/traditional-ai-vs-generative-ai
https://www.synopsys.com/blogs/chip-design/ai-chip-architecture.html
https://developer.ibm.com/articles/cc-machine-learning-deep-learning-architectures/
#NeuroSymbolicAI #AIInnovation #MachineLearning #SymbolicReasoning #ArtificialIntelligence #ExplainableAI #AIEthics #FutureOfAI #DeepLearning #CognitiveComputing #AIApplications #IntelligentSystems #AIResearch #RoboticIntelligence #NaturalLanguageProcessing #AutonomousSystems #AIInHealthcare #FinancialAI #AIEthics #TechInnovation