
Ten Groundbreaking Applications for Neuro-Symbolic AI (NSAI)
Oct 11
5 min read
0
4
0
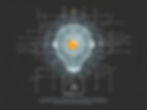
Introduction
Artificial Intelligence (AI) has come a long way since its inception, and one of the most exciting developments in recent years is Neuro-Symbolic AI (NSAI), which recently "won" a Nobel Prize (read below). This innovative approach combines the power of neural networks with symbolic reasoning, creating a hybrid system that promises to overcome many limitations of traditional AI. In this article, we'll explore ten compelling use cases for NSAI, demonstrating its potential to transform various industries and solve complex problems that have long challenged conventional AI systems.
Also see:
The Rise of Neuro-Symbolic AI: A Paradigm Shift in Artificial Intelligence
Achieving Advanced AI by Fusing Neuro-Symbolic AI & Hyperdimensional Computing
By the Way... Neuro-Symbolic AI Just "Won" a Nobel Prize
In a groundbreaking moment for the field of AI, the 2024 Nobel Prize in Physics was awarded to Geoffrey Hinton and John Hopfield for their foundational work in machine learning and artificial neural networks. This recognition highlights the growing importance of AI in scientific research and its potential to revolutionize various fields. While Hinton's work has primarily focused on deep learning, the award also acknowledges the significance of neurosymbolic approaches, as exemplified by Hopfield's contributions to associative memory networks.
Neuro-Symbolic AI (NSAI) Defined
Neuro-Symbolic AI (NSAI) is an advanced approach that integrates neural networks, which excel at pattern recognition and learning from data, with symbolic AI, which focuses on logic-based reasoning and knowledge representation. This combination allows NSAI systems to leverage the strengths of both approaches, resulting in AI that can learn from data, reason about abstract concepts, and explain its decision-making process.
History
The roots of NSAI can be traced back to the early days of AI research when scientists recognized the need to combine the strengths of neural networks and symbolic reasoning. John Hopfield's work on associative memory networks in the 1980s laid important groundwork for this approach. Over the years, researchers have continued to refine and develop NSAI techniques, leading to significant breakthroughs in recent years.
How is Neuro-Symbolic AI (NSAI) Not Like Your "Regular" AI?
Unlike traditional AI systems that rely solely on either neural networks or symbolic reasoning, NSAI combines both approaches. This integration allows NSAI to overcome limitations of each method when used in isolation. For example, while neural networks excel at pattern recognition, they often struggle with abstract reasoning and explainability. Symbolic AI, on the other hand, is good at logical reasoning but lacks the ability to learn from unstructured data. NSAI bridges this gap, creating AI systems that can both learn from data and reason about complex concepts.
"Unlike traditional AI systems that rely solely on either neural networks or symbolic reasoning, NSAI combines both approaches. This integration allows NSAI to overcome limitations of each method when used in isolation."
Ten Real-World and Possible Applications
Now, let's explore ten compelling use cases for Neuro-Symbolic AI (NSAI):
Medical Diagnosis and Treatment Planning: NSAI can analyze patient data, medical images, and scientific literature to assist doctors in making accurate diagnoses and developing personalized treatment plans. By combining pattern recognition with medical knowledge, NSAI can identify subtle indicators of diseases and suggest optimal treatment strategies. See How Zscale Labs™ uses NSAI for multi-label Chest X-Ray classification..
Financial Fraud Detection: In the finance sector, NSAI can detect fraudulent activities by analyzing transaction patterns while also considering complex rules and regulations. This approach can significantly reduce false positives and adapt to new fraud tactics more effectively than traditional methods.
Autonomous Vehicles: NSAI can enhance the decision-making capabilities of self-driving cars by combining real-time sensor data analysis with rule-based reasoning about traffic laws and safety protocols. This results in safer and more adaptable autonomous vehicles.
Natural Language Understanding: By integrating neural networks for language processing with symbolic knowledge representation, NSAI can achieve a deeper understanding of context, nuance, and abstract concepts in human language. This can lead to more sophisticated chatbots, virtual assistants, and language translation systems.
Drug Discovery: NSAI can accelerate the drug discovery process by analyzing molecular structures, predicting drug interactions, and reasoning about biological pathways. This approach can significantly reduce the time and cost associated with developing new medications.
Supply Chain Optimization: In logistics and supply chain management, NSAI can optimize routes, inventory levels, and resource allocation by combining data-driven predictions with rule-based constraints and business logic.
Cybersecurity: NSAI can enhance threat detection and response by analyzing network traffic patterns while also reasoning about known vulnerabilities, attack vectors, and security policies. This approach can lead to more robust and adaptive cybersecurity systems.
Robotics: In industrial and service robotics, NSAI can improve robot perception, decision-making, and task planning by combining visual and sensor data processing with symbolic reasoning about objects, environments, and task requirements.
Climate Modeling and Prediction: NSAI can enhance climate models by integrating data-driven analysis of weather patterns with symbolic reasoning about complex climate systems and physical laws. This can lead to more accurate long-term climate predictions and help in developing effective mitigation strategies.
Personalized Education: In the field of education, NSAI can create adaptive learning systems that combine pattern recognition of student behavior and performance with reasoning about educational concepts and learning strategies. This can result in highly personalized and effective learning experiences for students.
How Does Zscale Labs™ Use Neuro-Symbolic AI (NSAI)?
Zscale Labs™ is at the forefront of NSAI research and application. The company leverages NSAI in conjunction with Hyperdimensional Computing (HDC) to develop advanced AI solutions across various industries. One notable application is in medical imaging, where Zscale Labs™ has introduced Neuromorphic AI for multi-label Chest X-Ray classification.
This technology combines neural networks with symbolic reasoning to enhance diagnostic accuracy and efficiency in healthcare settings.
Read more here at Zscale Labs™
Five Related Technologies
Knowledge Graphs: These structured representations of information play a crucial role in NSAI by providing a framework for symbolic reasoning and knowledge integration.
Explainable AI (XAI): NSAI's ability to combine neural networks with symbolic reasoning aligns well with XAI goals, making AI decision-making processes more transparent and interpretable.
Transfer Learning: This technique, which allows AI models to apply knowledge gained from one task to another, complements NSAI's ability to integrate different types of knowledge and reasoning.
Reinforcement Learning: When combined with NSAI, reinforcement learning can create more adaptive and intelligent agents capable of learning from their environment while reasoning about complex goals and constraints.
Quantum Computing: As quantum computing technology advances, it has the potential to significantly enhance the computational capabilities of NSAI systems, enabling them to tackle even more complex problems.
Future Development & Challenges for Neuro-Symbolic AI (NSAI)
While NSAI shows great promise, several challenges need to be addressed for its continued development and widespread adoption:
Integration Complexity: Effectively combining neural networks and symbolic reasoning remains a significant technical challenge, requiring ongoing research and innovation.
Data Quality and Availability: NSAI systems require high-quality data for both their neural and symbolic components, which can be challenging to obtain in some domains.
Standardization: Developing standards and best practices for NSAI development and deployment will be essential for widespread adoption across industries.
Conclusion
Neuro-Symbolic AI represents a significant leap forward in the field of artificial intelligence, offering a powerful approach to solving complex problems that have long challenged traditional AI systems. By combining the strengths of neural networks and symbolic reasoning, NSAI has the potential to transform various industries and push the boundaries of what AI can achieve. As researchers and companies like Zscale Labs continue to innovate in this field, we can expect to see even more exciting applications and breakthroughs in the coming years. The recent Nobel Prize recognition further underscores the importance and potential of this technology, setting the stage for a new era of AI-driven innovation and discovery.
***
Join the LinkedIn Hyperdimensional Computing (HDC) Group! https://www.linkedin.com/groups/14521139/
***
References:
• https://en.wikipedia.org/wiki/Neuro-symbolic_AI
• https://www.techtarget.com/searchenterpriseai/definition/neuro-symbolic-AI
• https://www.linkedin.com/pulse/what-neuro-symbolic-artificial-intelligence-why-does-make-rouse
• https://www.medicaldevice-developments.com/news/zscale-labs-launches-neuromorphic-ai/
• https://www.zscalelabs.com/why-choose-us
• https://startupkitchen.community/neuro-symbolic-ai-why-is-it-the-future-of-artificial-intelligence/
• https://tdwi.org/Articles/2024/04/08/ADV-ALL-Can-Neuro-Symbolic-AI-Solve-AI-Weaknesses.aspx
• https://allegrograph.com/what-is-neuro-symbolic-ai/
• https://research.ibm.com/topics/neuro-symbolic-ai
• https://garymarcus.substack.com/p/two-nobel-prizes-for-ai-and-two-paths
• https://www.nytimes.com/2024/10/09/science/nobel-prize-chemistry.html
#NeuroSymbolicAI #AIRevolution #MachineLearning #SymbolicReasoning #MedicalDiagnosis #FinancialFraud #AutonomousVehicles #NLP #DrugDiscovery #SupplyChain #Cybersecurity #Robotics #ClimateModeling #PersonalizedEducation #KnowledgeGraphs #ExplainableAI #TransferLearning #ReinforcementLearning #QuantumComputing #AIEthics #ZscaleLabs #NeuroSymbolicAI #AI #NSAI #NeuromorphicAI #HyperdimensionalComputing #HDC